ANOVA: F-Ratio Testhttps://www.youtube.com/watch?v=51QZa7b0Ozk
A second indicator of how well the experimental data fits the regression model is an Analysis of Variance (ANOVA). Having determined an empirical model from prototype tests, the problem exists to determine whether the solution is believable and worth using. To consider this question, a sensitivity analysis is in order.
ANOVA is a technique for decomposing the total variation observed across experiments into sources of variation. The sources of variation are the important variables to consider; the variables that have no effect on the variation do not affect the product performance. ANOVA also has another important use: The total variability of the experiment can be quantified. Based on this quantification, the error variation in the model can be compared to the controlled variation of product design variables. Thus, the quality of an experimental model can be quantified
Theory
ANOVA is a method of analyzing the variation in response across an entire data set by decomposing the variation into the possible sources: the design variable changes and the experimental error. Thus ANOVA can be used to judge the importance of the different effects in a model and the quality of the model.
First we define some terminology. The overall average response (sometimes called the grand mean) for the entire set of experiments is denoted by:

Note this result is equivalent to β0.
Now, if the same test is repeated with the same design variable arrangement , in general the same value will not result, due to measuring error or uncontrolled noise. Assume for this analysis that we repeat r measurements at each .The average response at dh is:

where yhj is the jth replicate experiment using .
Recall our terminology, where we denote
• r as the number of replicates
• N as the number of different experimental arrangements .
Note that these variables are related by:
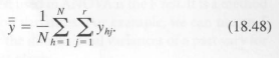
Derivation
Consider a decomposition of the total variation SS (sum of squares) into sources due to
• the mean
• to variation
• variation at a fixed (replication error)
We will ignore interaction effects explicitly in this derivation, but its extension is similar. With this assumption, we can model the response as:
or in words: average + to variations + fixed variations. The first term is the grand mean of the experiments, the second term is the effects due to changes in the design variables, and the last term is the inner error, due to experimental replication noise.
Date: 2016-01-14; view: 902
|